Transforming Channel Data into Predictive Insights

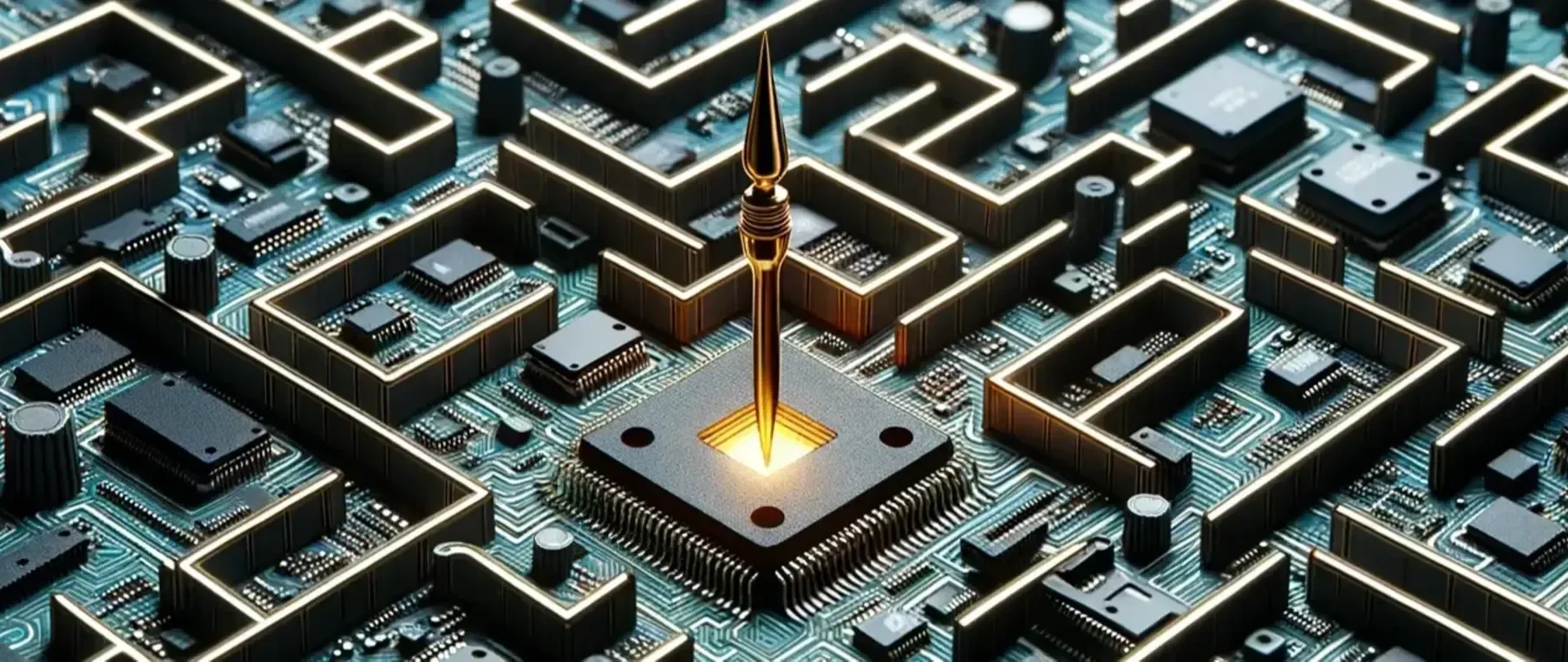

Navigating the intricate maze of hardware manufacturing logistics can be daunting, even for the most experienced operations managers. The journey from product conception to consumer's hands often spans 18-24 months, and the market structure's complexity makes understanding consumer demand akin to finding a needle in a haystack.
In an ideal scenario, components would travel directly from manufacturers to distributors, then onto system integrators, before finally reaching consumers. However, the reality is far more complex. Components often change hands multiple times, making it challenging to gauge market demand accurately. Original Equipment Manufacturers (OEMs) grapple with incomplete and inconsistent data.
Channel Data: A Potential Solution?
Channel data, specifically Point of Sale and Inventory data, is often seen as a potential solution. This data is invaluable for forecasting and closing the books each quarter, especially in programs where manufacturers offer incentives to their channel partners. However, obtaining this data from channel partners is fraught with challenges, including inconsistent data formats and a lack of expertise or resources to manage this complexity.
Current Approaches: A Dilemma
Manufacturers are currently faced with two less-than-ideal options. The first is to request "clean data" from partners, which often results in resistance as it shifts the burden onto the partners. The second option is to handle the inconsistency in-house, leading to high engineering costs and many unique scenarios. Both approaches are unsustainable and prone to errors, undermining confidence in the data.
Introducing PETL: A Game-Changer
PETL offers a novel solution to this problem. Designed to handle initial data transformations, PETL normalizes various partner submissions into a consistent format. It also extracts relevant information from unstructured text submitted by vendors.
At the core of PETL is The Protege Engine, a machine-learning feedback loop trained by the OEM's in-house experts. This allows for a constantly evolving system that can adapt to your channel data's unique needs and challenges.
Unique Features of PETL
PETL offers several unique features that simplify the process of managing channel data:
- Column Matching: Simplifies the onboarding process for new vendors by handling the initial setup and matching of input columns to normalized columns.
- Columnar Normalization: Corrects data in real-time, such as reformatting dates or currency amounts.
- Columnar Extraction: Uses custom-trained machine learning models to extract essential information like form factors, OEM names, Part SKUs, and Bar Codes from product descriptions.
- Columnar Validation: Ensures that all data fit into a set of predefined constraints and communicates in real-time with the partner for any needed corrections.
- Automated Feedback and Corrections: Drafts correspondence with the vendor to facilitate quick resubmission when machine learning falls short.
Transforming Data Stewardship Into Your Predictive Advantage
Implementing PETL does more than bring order to your data. It transforms raw, often inconsistent data into a robust analytical asset that can drive your business forward. Here's how:
Vendor Performance Analysis: Evaluate supply consistency and lead times to optimize your supply chain operations.
Product Life-Cycle Tracking: Make timely decisions on promotions, discontinuations, or revamps with real-time data.
Inventory Turnover Insights: Manage your inventory more efficiently by understanding which products are selling well and which are not.
Customer Segmentation: Identify high-value customer demographics for targeted marketing campaigns.
Market Basket Analysis: Improve your cross-selling and up-selling strategies by recognizing frequently co-purchased items.
Geographic Performance Metrics: Allocate resources more effectively by understanding where your products are performing well and where they aren't.
Heuristic Data Correction: Boost the accuracy of text analytics and NLP operations with our next-generation "Spell-Checker," which uses contextual understanding to correct text-based fields.
Machine Learning Problem-Solving Skillset: PETL employs adaptive machine learning algorithms that learn and adapt to new data scenarios, turning your data stewardship into a predictive, rather than reactive, operation.
Reinforcement Learning Human Feedback (RLHF) Loop: Improve the machine learning model's accuracy over time by flagging ambiguous cases for human review, bridging the gap between machine speed and human nuance.
Unlock Your Data's Full Potential with PETL
It's time to move beyond the limitations of traditional ETL solutions and embrace the future with PETL. With our cutting-edge technology, you're not just solving immediate problems but investing in a scalable, adaptable solution for all your data stewardship needs.
Don't leave your success to chance. Make informed decisions backed by data you can trust. Contact our sales team today and discover how PETL can make your data work for you.