Data Extraction for Hardware Manufacturers

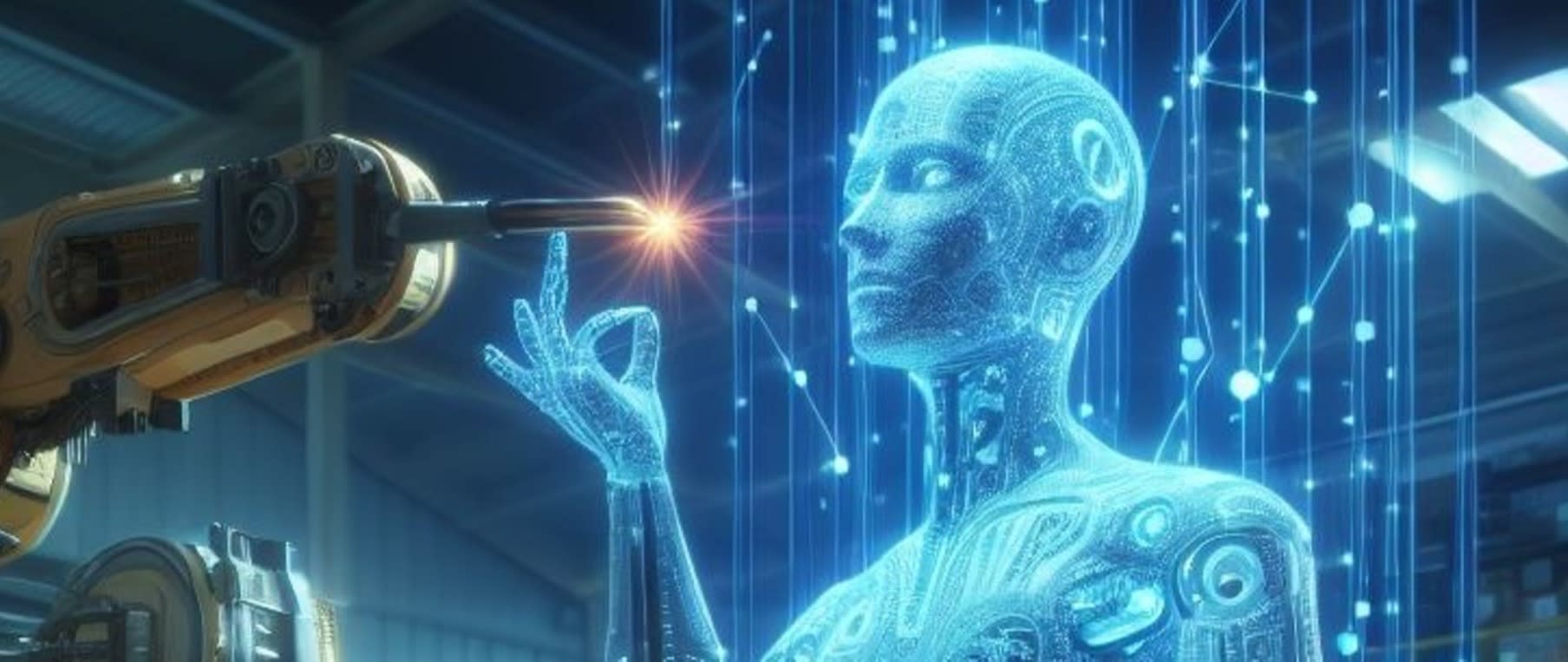

In the realm of hardware manufacturing, data extraction plays a pivotal role in decision-making. Granular insights can lead to significant business choices. However, this task is often more complex than it appears. Enter Protege Engine, a tool that revolutionizes data extraction capabilities with minimal setup. This post is designed for decision-makers in hardware manufacturing firms and tech enthusiasts interested in innovative data solutions.
Protege Extraction - The Basics
In the fast-paced world of hardware manufacturing, navigating the chaotic landscape of raw data is a common challenge. Tight schedules and the pressure to make informed choices mean we can't afford to wait for pristine data sets. Protege Engine is designed to address this issue. With just a few initial examples, our system can automate complex data extraction tasks, making your journey from raw data to actionable insights faster and more reliable.
The Challenge: Channel Partner Data and Its Transformation
In hardware manufacturing, the data you need often comes in fragments from various channel partners. For example, a GPU manufacturer might need to know which types of RAM their GPUs are commonly sold with. Similarly, a computer reseller might wish to understand the breakdown of CPUs moving through their sales channels. Each of these insights informs sales forecasting, product development, and other key business decisions.
The ETL Dilemma: Product Descriptions and System Variability
The Extraction, Transformation, and Loading (ETL) process involves handling data from as many inventory and Point-of-Sale (PoS) systems as you have channel partners. More often than not, product descriptions become jumbled strings of text—concatenated and delimited by various symbols.
Language and Format Barriers
These descriptions can often be in non-ASCII languages like Chinese or Russian, or laden with acronyms and industry jargon. Non-ASCII languages are those that use characters not included in the American Standard Code for Information Interchange (ASCII), such as Chinese or Russian. The prospect of writing code that can adapt to this myriad of formats is not just daunting; it's practically impossible.
The Protege Engine Solution
Traditional large-scale language models often fall short in specialized, domain-specific needs like hardware manufacturing. This is where Protege Engine utilizes its groundbreaking Reinforcement Learning from Human Feedback (RLHF) system.
The RLHF Paradigm
RLHF is a system that integrates human expertise directly into ongoing model training, creating a dynamic feedback loop. This allows Protege Engine to adapt and evolve rapidly, gaining the domain-specific knowledge necessary for managing complex data, such as hardware product descriptions.
The Power and Efficiency of Protege Engine
The Protege Engine leverages RLHF to generate synthetic training data at a fast pace. This fuels smaller, more specialized models that offer top-notch performance at a fraction of the cost—between 150x to 200x cheaper than the alternatives.
Real-World Application of Protege Engine in Data Extraction
In the context of hardware manufacturing and Partner Channel Sales Data, Protege Engine excels at tasks like Product Type Identification and System Description Extraction. By learning from human experts in real time, Protege Engine offers an unparalleled advantage in automating and enhancing data extraction processes.
Protege Engine is a game-changer in the data-driven landscape of hardware manufacturing. Its agile, cost-effective, and specialized capabilities make it the go-to choice for extracting and interpreting complex data. Interested in learning more? Schedule a demo or consultation today to see how we can elevate your data stewardship journey.