Protege Engine: Cost-Effective AI Through Human Experience

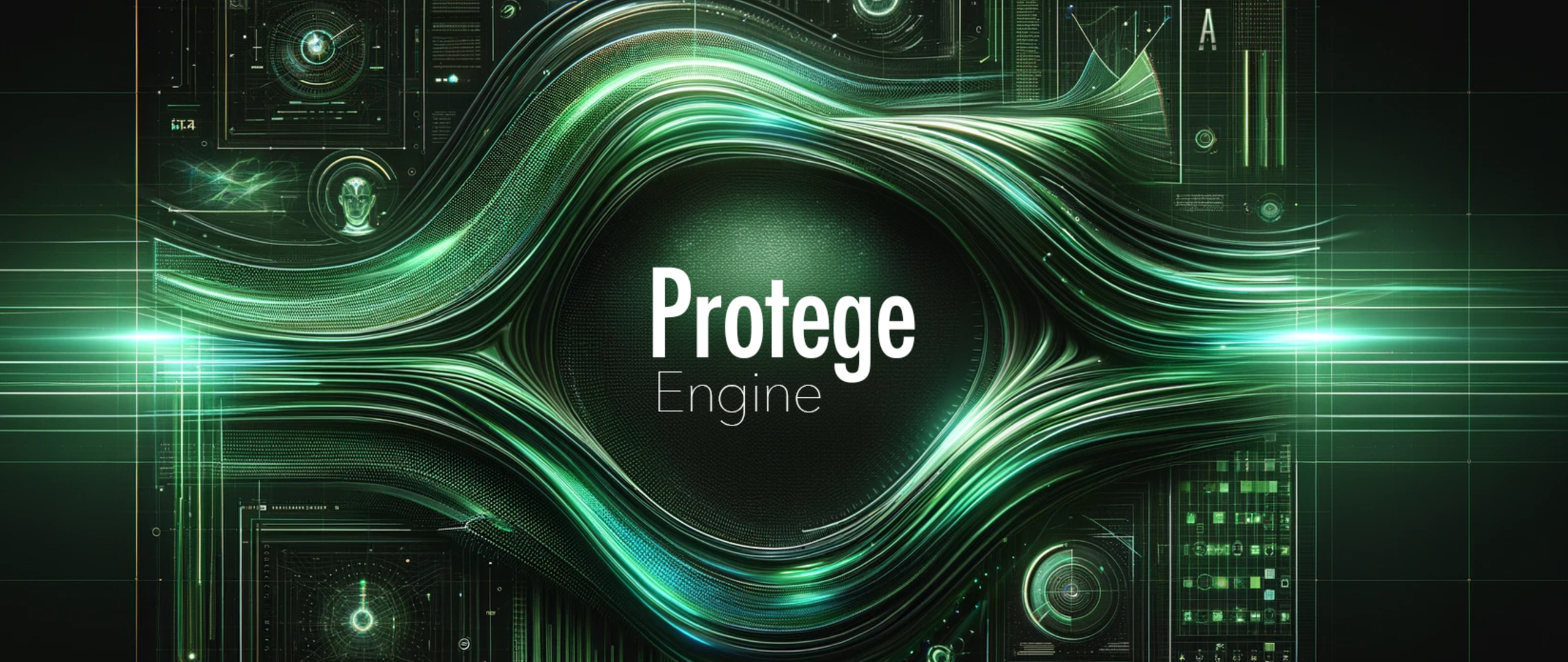

Introduction
In an era awash with promises of AI’s transformative power, the gap between potential and practical application remains vast. While large language models like GPT-4 grab headlines, they often falter when applied to specialized tasks in heavy and tech industries. This is where the Protege Engine shines. Leveraging our groundbreaking Reinforcement Learning Human Feedback (RLHF) loop, we’re setting new standards in data stewardship services, delivering precision and cost-efficiency.
The Challenge of Specialized AI
Broad-scope models from tech giants such as OpenAI and Google are remarkable but often lack the granularity required for domain-specific applications. The notion of “training” these behemoths conjures a litany of challenges. The fundamental principle of garbage in, garbage out still applies; if your training data isn't up to scratch, neither will your outcomes.
The RLHF Advantage
What sets RLHF apart is its unique ability to weave human expertise seamlessly into the machine learning model's training fabric. Instead of relying solely on pre-labeled data sets, RLHF uses a continual feedback loop, driven by human interaction, to refine the model’s understanding and performance.
The Prowess of the Protege Engine
Our Protege Engine embodies RLHF to generate synthetic training data at an unparalleled pace. This rapid data generation feeds into smaller, but impressively efficient models. What's the kicker? These highly specialized models not only rival GPT-4 in performance but do so at a cost that's between 150x to 200x cheaper.
Harnessing Human Feedback
Our system embraces two types of human feedback to enrich the RLHF loop:
Explicit Feedback: Think of this as the AI equivalent of Google’s RECAPTCHA, where users directly validate or correct the model's predictions.
Implicit Feedback: This is subtler but equally valuable. As users interact with the integrated product, their modifications, corrections, or even new labels become invaluable feedback for ongoing training.
Real-World Applications
Our RLHF approach has already proven its mettle in managing Partner Channel Sales Data for hardware manufacturers. Specifically, tasks like:
Product Type Identification: categorizes products into nuanced classes such as computer systems, parts, services, or software.
System Description Extraction: parses unstructured text to identify a computer system's key components.
The Business Impact
When your sales team are experts but don't have the time to annotate each piece of data manually, our system offers a unique advantage. Protege Engine learns non-intrusively from experts as they carry out their regular tasks, fine-tuning itself in real time and supercharging your data automation processes.
Conclusion
Protege Engine is a transformative force in enterprise AI, proficiently converting human expertise into actionable machine learning models. If you aim to harness specialized "tribal knowledge" for model training, look no further. We offer the technology, proficiency, and cost-effectiveness to make it happen.
Next Steps
Ready to revolutionize your enterprise with human-informed AI? Reach out to us for a consultation.